tl;dr; Periodically, the whole of MDN is built, by our Node code, in a GitHub Action. A Python script bulk-publishes this to Elasticsearch. Our Django server queries the same Elasticsearch via /api/v1/search
. The site-search page is a static single-page app that sends XHR requests to the /api/v1/search
endpoint. Search results' sort-order is determined by match and "popularity".
Jamstack'ing
The challenge with "Jamstack" websites is with data that is too vast and dynamic that it doesn't make sense to build statically. Search is one of those. For the record, as of Feb 2021, MDN consists of 11,619 documents (aka. articles) in English. Roughly another 40,000 translated documents. In English alone, there are 5.3 million words. So to build a good search experience we need to, as a static site build side-effect, index all of this in a full-text search database. And Elasticsearch is one such database and it's good. In particular, Elasticsearch is something MDN is already quite familiar with because it's what was used from within the Django app when MDN was a wiki.
Note: MDN gets about 20k site-searches per day from within the site.
Build
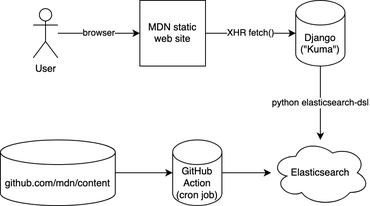
When we build the whole site, it's a script that basically loops over all the raw content, applies macros and fixes, dumps one index.html
(via React server-side rendering) and one index.json
. The index.json
contains all the fully rendered text (as HTML!) in blocks of "prose". It looks something like this:
{
"doc": {
"title": "DOCUMENT TITLE",
"summary": "DOCUMENT SUMMARY",
"body": [
{
"type": "prose",
"value": {
"id": "introduction",
"title": "INTRODUCTION",
"content": "<p>FIRST BLOCK OF TEXTS</p>"
}
},
...
],
"popularity": 0.12345,
...
}
You can see one here: /en-US/docs/Web/index.json
Indexing
Next, after all the index.json
files have been produced, a Python script takes over and it traverses all the index.json
files and based on that structure it figures out the, title, summary, and the whole body (as HTML).
Next up, before sending this into the bulk-publisher in Elasticsearch it strips the HTML. It's a bit more than just turning <p>Some <em>cool</em> text.</p>
to Some cool text.
because it also cleans up things like <div class="hidden">
and certain <div class="notecard warning">
blocks.
One thing worth noting is that this whole thing runs roughly every 24 hours and then it builds everything. But what if, between two runs, a certain page has been removed (or moved), how do you remove what was previously added to Elasticsearch? The solution is simple: it deletes and re-creates the index from scratch every day. The whole bulk-publish takes a while so right after the index has been deleted, the searches won't be that great. Someone could be unlucky in that they're searching MDN a couple of seconds after the index was deleted and now waiting for it to build up again.
It's an unfortunate reality but it's a risk worth taking for the sake of simplicity. Also, most people are searching for things in English and specifically the Web/
tree so the bulk-publishing is done in a way the most popular content is bulk-published first and the rest was done after. Here's what the build output logs:
Found 50,461 (potential) documents to index
Deleting any possible existing index and creating a new one called mdn_docs
Took 3m 35s to index 50,362 documents. Approximately 234.1 docs/second
Counts per priority prefixes:
en-us/docs/web 9,056
*rest* 41,306
So, yes, for 3m 35s there's stuff missing from the index and some unlucky few will get fewer search results than they should. But we can optimize this in the future.
Searching
The way you connect to Elasticsearch is simply by a URL it looks something like this:
https://USER:PASSWD@HASH.us-west-2.aws.found.io:9243
It's an Elasticsearch cluster managed by Elastic running inside AWS. Our job is to make sure that we put the exact same URL in our GitHub Action ("the writer") as we put it into our Django server ("the reader").
In fact, we have 3 Elastic clusters: Prod, Stage, Dev.
And we have 2 Django servers: Prod, Stage.
So we just need to carefully make sure the secrets are set correctly to match the right environment.
Now, in the Django server, we just need to convert a request like GET /api/v1/search?q=foo&locale=fr
(for example) to a query to send to Elasticsearch. We have a simple Django view function that validates the query string parameters, does some rate-limiting, creates a query (using elasticsearch-dsl
) and packages the Elasticsearch results back to JSON.
How we make that query is important. In here lies the most important feature of the search; how it sorts results.
In one simple explanation, the sort order is a combination of popularity and "matchness". The assumption is that most people want the popular content. I.e. they search for foreach
and mean to go to /en-US/docs/Web/JavaScript/Reference/Global_Objects/Array/forEach
not /en-US/docs/Web/API/NodeList/forEach
both of which contains forEach
in the title. The "popularity" is based on Google Analytics pageviews which we download periodically, normalize into a floating-point number between 1 and 0. At the of writing the scoring function does something like this:
rank = doc.popularity * 10 + search.score
This seems to produce pretty reasonable results.
But there's more to the "matchness" too. Elasticsearch has its own API for defining boosting and the way we apply is:
- match phrase in the
title
: Boost = 10.0
- match phrase in the
body
: Boost = 5.0
- match in
title
: Boost = 2.0
- match in
body
: Boost = 1.0
This is then applied on top of whatever else Elasticsearch does such as "Term Frequency" and "Inverse Document Frequency" (tf and if). This article is a helpful introduction.
We're most likely not done with this. There's probably a lot more we can do to tune this myriad of knobs and sliders to get the best possible ranking of documents that match.
Web UI
The last piece of the puzzle is how we display all of this to the user. The way it works is that developer.mozilla.org/$locale/search
returns a static page that is blank. As soon as the page has loaded, it lazy-loads JavaScript that can actually issue the XHR request to get and display search results. The code looks something like this:
function SearchResults() {
const [searchParams] = useSearchParams();
const sp = createSearchParams(searchParams);
const fetchURL = `/api/v1/search?${sp.toString()}`;
const { data, error } = useSWR(
fetchURL,
async (url) => {
const response = await fetch(URL);
return await response.json();
}
);
A lot of interesting details are omitted from this code snippet. You have to check it out for yourself to get a more up-to-date insight into how it actually works. But basically, the window.location
(and pushState
) query string drives the fetch()
call and then all the component has to do is display the search results with some highlighting.
The /api/v1/search
endpoint also runs a suggestion query as part of the main search query. This extracts out interest alternative search queries. These are filtered and scored and we issue "sub-queries" just to get a count for each. Now we can do one of those "Did you mean...". For example: search for intersections
.
In conclusion
There are a lot of interesting, important, and careful details that are glossed over here in this blog post. It's a constantly evolving system and we're constantly trying to improve and perfect the system in a way that it fits what users expect.
A lot of people reach MDN via a Google search (e.g. mdn array foreach
) but despite that, nearly 5% of all traffic on MDN is the site-search functionality. The /$locale/search?...
endpoint is the most frequently viewed page of all of MDN. And having a good search engine that's reliable is nevertheless important. By owning and controlling the whole pipeline allows us to do specific things that are unique to MDN that other websites don't need. For example, we index a lot of raw HTML (e.g. <video>
) and we have code snippets that needs to be searchable.
Hopefully, the MDN site-search will elevate from being known to be very limited to something now that can genuinely help people get to the exact page better than Google can. Yes, it's worth aiming high!