This an optimization story that should not surprise anyone using the Django ORM. But I thought I'd share because I have numbers now! The origin of this came from a real requirement. For a given parent model, I'd like to extract the value of the name
column of all its child models, and the turn all these name
strings into 1 MD5 checksum string.
Variants
The first attempted looked like this:
artist = Artist.objects.get(name="Bad Religion")
names = []
for song in Song.objects.filter(artist=artist):
names.append(song.name)
return hashlib.md5("".join(names).encode("utf-8")).hexdigest()
The SQL used to generate this is as follows:
SELECT "main_song"."id", "main_song"."artist_id", "main_song"."name",
"main_song"."text", "main_song"."language", "main_song"."key_phrases",
"main_song"."popularity", "main_song"."text_length", "main_song"."metadata",
"main_song"."created", "main_song"."modified",
"main_song"."has_lastfm_listeners", "main_song"."has_spotify_popularity"
FROM "main_song" WHERE "main_song"."artist_id" = 22729;
Clearly, I don't need anything but just the name
column, version 2:
artist = Artist.objects.get(name="Bad Religion")
names = []
for song in Song.objects.filter(artist=artist).only("name"):
names.append(song.name)
return hashlib.md5("".join(names).encode("utf-8")).hexdigest()
Now, the SQL used is:
SELECT "main_song"."id", "main_song"."name"
FROM "main_song" WHERE "main_song"."artist_id" = 22729;
But still, since I don't really need instances of model class Song
I can use the .values()
method which gives back a list of dictionaries. This is version 3:
names = []
for song in Song.objects.filter(artist=a).values("name"):
names.append(song["name"])
return hashlib.md5("".join(names).encode("utf-8")).hexdigest()
This time Django figures it doesn't even need the primary key value so it looks like this:
SELECT "main_song"."name" FROM "main_song" WHERE "main_song"."artist_id" = 22729;
Last but not least; there is an even faster one. values_list()
. This time it doesn't even bother to map the column name to the value in a dictionary. And since I only need 1 column's value, I can set flat=True
. Version 4 looks like this:
names = []
for name in Song.objects.filter(artist=a).values_list("name", flat=True):
names.append(name)
return hashlib.md5("".join(names).encode("utf-8")).hexdigest()
Same SQL gets used this time as in version 3.
The benchmark
Hopefully this little benchmark script speaks for itself:
from songsearch.main.models import *
import hashlib
def f1(a):
names = []
for song in Song.objects.filter(artist=a):
names.append(song.name)
return hashlib.md5("".join(names).encode("utf-8")).hexdigest()
def f2(a):
names = []
for song in Song.objects.filter(artist=a).only("name"):
names.append(song.name)
return hashlib.md5("".join(names).encode("utf-8")).hexdigest()
def f3(a):
names = []
for song in Song.objects.filter(artist=a).values("name"):
names.append(song["name"])
return hashlib.md5("".join(names).encode("utf-8")).hexdigest()
def f4(a):
names = []
for name in Song.objects.filter(artist=a).values_list("name", flat=True):
names.append(name)
return hashlib.md5("".join(names).encode("utf-8")).hexdigest()
artist = Artist.objects.get(name="Bad Religion")
print(Song.objects.filter(artist=artist).count())
print(f1(artist) == f2(artist))
print(f2(artist) == f3(artist))
print(f3(artist) == f4(artist))
import time
import random
import statistics
functions = f1, f2, f3, f4
times = {f.__name__: [] for f in functions}
for i in range(500):
func = random.choice(functions)
t0 = time.time()
func(artist)
t1 = time.time()
times[func.__name__].append((t1 - t0) * 1000)
for name in sorted(times):
numbers = times[name]
print("FUNCTION:", name, "Used", len(numbers), "times")
print("\tBEST", min(numbers))
print("\tMEDIAN", statistics.median(numbers))
print("\tMEAN ", statistics.mean(numbers))
print("\tSTDEV ", statistics.stdev(numbers))
I ran this on my PostgreSQL 11.1 on my MacBook Pro with Django 2.1.7. So the database is on localhost
.
The results
276
True
True
True
FUNCTION: f1 Used 135 times
BEST 6.309986114501953
MEDIAN 7.531881332397461
MEAN 7.834429211086697
STDEV 2.03779968066591
FUNCTION: f2 Used 135 times
BEST 3.039121627807617
MEDIAN 3.7298202514648438
MEAN 4.012803678159361
STDEV 1.8498943539073027
FUNCTION: f3 Used 110 times
BEST 0.9920597076416016
MEDIAN 1.4405250549316406
MEAN 1.5053835782137783
STDEV 0.3523240470133114
FUNCTION: f4 Used 120 times
BEST 0.9369850158691406
MEDIAN 1.3251304626464844
MEAN 1.4017681280771892
STDEV 0.3391019435930447
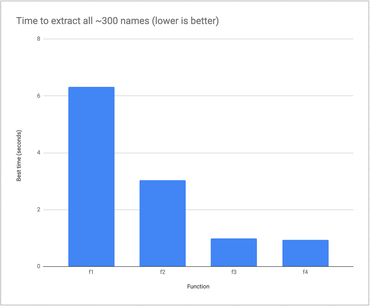
Discussion
I guess the hashlib.md5("".join(names).encode("utf-8")).hexdigest()
stuff is a bit "off-topic" but I checked and it's roughly 300 times faster than building up the names
list.
It's clearly better to ask less of Python and PostgreSQL to get a better total time. No surprise there. What was interesting was the proportion of these differences. Memorize that and you'll be better equipped if it's worth the hassle of not using the Django ORM in the most basic form.
Also, do take note that this is only relevant in when dealing with many records. The slowest variant (f1
) takes, on average, 7 milliseconds.
Summarizing the difference with percentages compared to the fastest variant:
f1
- 573% slower
f2
- 225% slower
f3
- 6% slower
f4
- 0% slower
UPDATE Feb 25 2019
James suggested, although a bit "missing the point", that it could be even faster if all the aggregation is pushed into the PostgreSQL server and then the only thing that needs to transfer from PostgreSQL to Python is the final result.
By the way, name
column in this particular benchmark, when concatenated into one big string, is ~4KB. So, with variant f5
it only needs to transfer 32 bytes which will/would make a bigger difference if the network latency is higher.
Here's the whole script: https://gist.github.com/peterbe/b2b7ed95d422ab25a65639cb8412e75e
And the results:
276
True
True
True
False
False
FUNCTION: f1 Used 92 times
BEST 5.928993225097656
MEDIAN 7.311463356018066
MEAN 7.594626882801885
STDEV 2.2027017044658423
FUNCTION: f2 Used 75 times
BEST 2.878904342651367
MEDIAN 3.3979415893554688
MEAN 3.4774907430013022
STDEV 0.5120246550765524
FUNCTION: f3 Used 88 times
BEST 0.9310245513916016
MEDIAN 1.1944770812988281
MEAN 1.3105544176968662
STDEV 0.35922655625999383
FUNCTION: f4 Used 71 times
BEST 0.7879734039306641
MEDIAN 1.1661052703857422
MEAN 1.2262606284987758
STDEV 0.3561764250427344
FUNCTION: f5 Used 90 times
BEST 0.7929801940917969
MEDIAN 1.0334253311157227
MEAN 1.1836051940917969
STDEV 0.4001442703048186
FUNCTION: f6 Used 84 times
BEST 0.80108642578125
MEDIAN 1.1119842529296875
MEAN 1.2281338373819988
STDEV 0.37146893005516973
Result: f5
is takes 0.793ms and (the previous "winner") f4
takes 0.788ms.
I'm not entirely sure why f5
isn't faster but I suspect it's because the dataset is too small for it all to matter.
Compare:
songsearch=# explain analyze SELECT "main_song"."name" FROM "main_song" WHERE "main_song"."artist_id" = 22729;
QUERY PLAN
------------------------------------------------------------------------------------------------------------------------------------
Index Scan using main_song_ca949605 on main_song (cost=0.43..229.33 rows=56 width=16) (actual time=0.014..0.208 rows=276 loops=1)
Index Cond: (artist_id = 22729)
Planning Time: 0.113 ms
Execution Time: 0.242 ms
(4 rows)
with...
songsearch=# explain analyze SELECT md5(STRING_AGG("main_song"."name", '')) AS "names_hash" FROM "main_song" WHERE "main_song"."artist_id" = 22729;
QUERY PLAN
------------------------------------------------------------------------------------------------------------------------------------------
Aggregate (cost=229.47..229.48 rows=1 width=32) (actual time=0.278..0.278 rows=1 loops=1)
-> Index Scan using main_song_ca949605 on main_song (cost=0.43..229.33 rows=56 width=16) (actual time=0.019..0.204 rows=276 loops=1)
Index Cond: (artist_id = 22729)
Planning Time: 0.115 ms
Execution Time: 0.315 ms
(5 rows)
I ran these two SQL statements about 100 times each and recorded their best possible execution times:
1) The plain SELECT
- 0.99ms
2) The STRING_AGG
- 1.06ms
So that accounts from ~0.1ms difference only! Which kinda matches the results seen above. All in all, I think the dataset is too small to demonstrate this technique. But, considering the chance that the complexity might not be linear with the performance benefit, it's still interesting.
Even though this tangent is a big off-topic, it is often a great idea to push as much work into the database as you can if applicable. Especially if it means you can transfer a lot less data eventually.